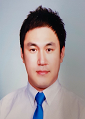
Ho Kim
Yonsei University, Seoul, South Korea
Title: Predictive Modeling for unmet needs in medical utilization by machine learning approach: using Korea health panel data 2011~2013 year
Biography
Biography: Ho Kim
Abstract
Unmet need for medical care in Korea has been increasing due to a lot of reasons such as social, econmic or mental status, which has been associated with health inequalities. Therefore, the aim of this study is to develop predictive models for unmet medical need using both logistic regression model and random forest machine-learning approach. Korea Health Panel data from 2011 to 2013 year, surveyed by Korea Institute for Health and Social Affairs and National Health Insrance Service, were used for this study. There were 38 variables including independent variables such as age, educational level, marital status, economic activity, hosehold income quintile, health insurance type, national pension participation status and others. Dependent variable refered to the experience of an unmet need in using medical service although being needed. Total observations in 12,256 subjects were 30,061 and devided into training (18,037:60%), validation (6,013:20%) and test (6,013:20%). Machine-learning approach with logistic regression model and random forest model were used to predict the unmet need. Overall, 16.2% (n=4,864) of observations experiened unmet need in using medical service even though they wanted or had to use it. The logistic regression model showed area under curve (AUC) as 0.696 and random forest model presented 0.937 AUC in training, validation and test data-set. Conclusivly, prediction result could be different based on which machine-learning approach would be used for unmet need for medical service. And AUC of random forest machine-learning approach is shown to be better than that of logistic regression model.